1 Introduction
Large language models (LLMs), relying on the Transformer architecture proposed by Vaswani
et al. (
2017) have shown remarkable effectiveness in many natural language processing (NLP) tasks (Minaee
et al.,
2024; Naveed
et al.,
2024). This has primarily been fuelled by increasingly large model parameterisations and training datasets, which are deemed essential according to neural scaling laws (Hernandez
et al.,
2022). On the other hand, with the consistent advancement of computational linguistics and NLP, open LLMs such as Llama2 (Touvron
et al.,
2023), Mistral (Jiang
et al.,
2023), Mixtral (Jiang
et al.,
2024), Falcon (Almazrouei
et al.,
2023) were released. The performance characteristics of these open models are comparable with their commercial counterparts. Such LLMs usually require massive datasets and considerable computational resources. For example, the pretraining of the Llama2 family was carried out using a 2 trillion token set and required 3311616 GPU hours (Touvron
et al.,
2023). In addition to direct applications, these models can be further trained for various downstream problems (Minaee
et al.,
2024), including regional language modelling.
For this application, it is important to note that open LLMs are usually trained with largely English texts (e.g. Touvron
et al. (
2023) indicate that
$\gt 89\% $ of the dataset, which was used to pretrain Llama2 consisted of English texts), resulting in a lack of performance for less common languages. Although commercial LLMs usually better support underrepresented languages, as a rule, they are exposed only via APIs, which do not provide access to the model’s parameters or its intermediate representations. Since there are
$\approx 380$ non-English languages with at least 1 million speakers (Eberhard
et al.,
2021), open regional LLMs constitute an important research direction and there have been multiple recent attempts to achieve efficient open LLMs, tailored for various regional languages (see Section
2, Table
1).
Regional LLM training is a challenging technical task not only computationally but also from the perspective of training data, which should reflect a rich structure of the language of interest, local cultural nuances, and domain-specific knowledge in multiple areas. Although this is only partially solved by massive multilingual datasets, such as CulturaX (Nguyen
et al.,
2023), it is still an important challenge to collect representative datasets in regional languages.
Open LLMs are also potentially useful for NLP research as their internal mechanism is fully transparent. There are also related applications outside the scope of NLP. For example, successful regional LLMs can significantly impact areas such as education, public services, healthcare, and cultural preservation.
This article describes Neurotechnology’s contribution to regional LLM research, consisting of
-
• Llama2-based 7 and 13 billion parameter LLMs for the Lithuanian language, and their empirical evaluation;
-
• A new dataset, consisting of 13,848 Q/A pairs primarily about Lithuania and Lithuanian history (in the Lithuanian language);
-
• Translations of popular LLM benchmarks to the Lithuanian language;
-
• Open repository, containing all the mentioned components.
In this article, we investigate whether efficient Lithuanian LLMs can be achieved from Llama2 LLMs (which do not have the Lithuanian component, according to Touvron
et al.,
2023). In our opinion, for this research the Llama2 architecture is potentially advantageous against other similar open LLMs without Lithuanian language support (e.g. Mistral), since it allows experimentation with different model sizes, and its 13 billion parameter version nearly matches the performance of Mistral, as shown by Jiang
et al. (
2023).
We structure our paper by starting with a short review of the related work in Section
2. Section
3 describes the proposed LLMs and other contributed components, and Section
4 is devoted to an empirical evaluation. Finally, the conclusive Section
5 summarises the research conducted from different perspectives.
2 Related Work
Llama2 model. Transformer-based Llama2 is available in different parameter sizes (e.g. 7, 13, and 70 billion parameters). The model is first pretrained using a 2 trillion token set, collected from public sources, and utilising a self-supervised autoregressive approach with cross-entropy loss. Afterward, it is fine-tuned using publicly available instruction datasets, augmented with human-annotated data, and Reinforcement Learning with Human Feedback (RLHF) methodologies (Touvron
et al.,
2023).
This model can support the maximum context length of the 4096 tokens. According to benchmarks, Llama2 generally performs on par with various open alternatives (e.g. Falcon (Almazrouei
et al.,
2023), Mistral (Jiang
et al.,
2023) and Mixtral (Jiang
et al.,
2024)), which also may be advantageous in specific scenarios. For example, Falcon is recognized for its strong performance at higher parameter counts, and Mistral/Mixtral are generally lighter-weight models that emphasize efficiency and specialized use cases. Compared to these models, Llama2 aims to maintain a balance between robust performance and scalability. As is common with large foundational models, it can be further successfully tuned for various downstream tasks, including regional language modelling.
LLMs for regional languages. Table
1 summarises LLMs tailored for common European languages, reflecting the recent contributions from the research and engineering community working in this direction. We include only those regional LLMs, that meet the following criteria:
-
• The model should be published in an open repository (e.g. Hugging Face),
-
• It should contain at least a minimal description (architecture, training data, and other details).
According to Table
1, open LLMs are released for the majority of common European languages. Table
1 shows that Llama2 and Mistral are the leading architectures for open LLMs for regional European languages, and 7 billion parameter models are the most common. Table
1 also reveals that full parameter training is conducted in the majority of cases (19 cases from 20), instead of the parameter-efficient fine-tuning (PEFT) based approach. However, in some instances (2 cases from 20) regional LLMs were trained using PEFT methods, such as LoRA (Hu
et al.,
2022), which may result in less accurate models compared to full-parameter training, although with the lower computational costs. In addition, quite often only the model itself is published (11 out of 20 cases), without an accompanying citable document (e.g. technical report/peer-reviewed publication), or training and evaluation datasets. In our opinion, the lack of accompanying scientific documentation limits the potential usefulness of the released regional LLMs in various important aspects, including their reproducibility, empirical performance assessment, and establishing a connection to the existing related results.
Multilingual LLMs that support the Lithuanian language. Another way of achieving LLMs with regional language support is to train models for multiple languages simultaneously. Although this approach requires much more computational and data resources (for instance, EuroLLM was pretrained using 256 Nvidia H100 GPU’s and 4 trillion token set, as indicated by Martins
et al.,
2024), compared to learning models only for single language, recent open LLMs that support Lithuanian language are multilingual (e.g. Llama3.X (Grattafiori
et al.,
2024), and Gemma2 (Riviere and et al.,
2024), and EuroLLM). Although these LLMs perform quite similarly on various benchmarks, there are applications in which some of these models are advantageous against other counterparts. For example, Gemma2 is potentially more suitable for general knowledge and reasoning tasks, Llama3.1 is efficient in coding and complex problem-solving tasks, and EuroLLM is optimised for European languages. All these multilingual models with Lithuanian language support were published in later stages or after our research and currently represent state-of-the-art (SOTA) in the field of open LLMs.
Table 1
Open LLM models for regional European languages. The F/P column denotes whether the model was full-parameter trained (F), or trained via PEFT (P), and Doc. column shows whether the corresponding model has an accompanying publication.
Language and reference |
Architecture |
Size |
F/P |
Doc. |
Bulgarian (INSAIT, 2024) |
Mistral |
7B |
F |
No |
Danish (Mabeck, 2024) |
Mistral |
7B |
F |
No |
Dutch (Rijgersberg, 2024) |
Mistral |
7B |
F |
No |
French-English (Faysse et al., 2024) |
Llama |
1.3B |
F |
Yes |
German (Plüster and Schuhmann, 2024) |
Llama2 |
7B,13B |
F |
No |
Greek (SPAHE, 2024) |
Mistral |
7B |
F |
No |
Hungarian-English (Csaki et al., 2024) |
Llama2 |
7B |
F |
Yes |
Finnish and other (LumiOpen, 2024) |
Llama2 |
7B–33B |
F |
No |
Icelandic (Snæbjarnarson et al., 2022) |
RoBERTa |
|
F |
Yes |
Italian (Bacciu et al., 2023) |
Llama2 |
7B,13B |
P |
Yes |
Lithuanian (Ours) |
Llama2 |
7B,13B |
F |
Yes |
Norwegian (Norallm, 2024) |
Mistral |
7B |
F |
No |
Serbian, Bosnian, Croatian (Gordić, 2024) |
Mistral |
7B |
F |
No |
Spanish (Projecte AINA, 2024) |
Falcon |
7B |
F |
No |
Swedish (Ekgren et al., 2023) |
GPT-SW3 |
126M–40B |
F |
Yes |
Slovenian (Ulčar and Robnik-Šikonja, 2021) |
RoBERTa |
|
F |
Yes |
Polish (Speakleash, 2024) |
Mistral |
7B |
F |
No |
Ukrainian (Boros et al., 2024) |
Mistral |
7B |
F |
Yes |
Portuguese (Garcia et al., 2024) |
Phi-2B |
1.3B–7B |
P |
Yes |
Romanian (Masala et al., 2024) |
Llama2 |
7B |
F |
Yes |
3 Proposed Open LLMs and Accompanying Components
Proposed open LLMs and their training details. We trained the proposed LLMs (including tokenizers) from Llama2-7B and Llama2-13B, respectively (Table
2).
Table 2
Overview of Llama-based LLMs.
Model name |
Description |
Llama2-7B |
A second-generation Llama foundational language model with 7 billion parameters (no Lithuanian language support).
|
LT-Llama2-7B |
Proposed Lithuanian LLM derived from Llama2-7B model, according to information, provided in Section3. |
Llama2-13B |
A second-generation Llama foundational language model with 13 billion parameters (no Lithuanian language support).
|
LT-Llama2-13B |
Proposed Lithuanian LLM derived from Llama2-13B model, according to information, provided in Section3. |
The training follows a standard two-step approach, consisting of autoregressive pretraining and supervised fine-tuning, which schematically is depicted in Fig.
1.
Autoregressive pretraining was performed on the Lithuanian component of the CulturaX dataset (Nguyen
et al.,
2023). It is the most intensive step computationally (Table
3), and corresponds to the integration of the Lithuanian language into the model. During this step, the cross-entropy loss for the next token prediction task was minimised (hence, no labelled data are required for pretraining). The complete set of model’s parameters was optimised (i.e. no PEFT was used). Figure
2 shows the loss during the pretraining process. From it we see that, although loss minimisation tends to saturate in the end, one may hypothesise that the learning would continue for more than one epoch.
Figure
6 (Appendix
A) shows the distribution of the source of the Lithuanian component of the CulturaX dataset. From it we see that this dataset is quite rich in quantity. However, it is collected mainly from common web sites. Figure
7 (Appendix
A) shows the distribution of the length of the record in tokens. In order to speed up pretraining, we reduced the context length to 2048 tokens (reflected in the peak near 2048, in Fig.
7 (Appendix
A)). See Table
3 for more details on the pretraining process.
Supervised fine-tuning (SFT) explicitly guides the pretrained model toward task-specific outputs using labelled data. It is much less computationally intensive, since the model already has the Lithuanian language integrated (Table
3). We conducted SFT using the Alpaca dataset (Dubois
et al.,
2024), which has been translated into Lithuanian using the ChatGPT (gpt-4-1106-preview) and dataset (Neurotechnology,
2024). SFT tunes the LLMs to process formatted prompts
"[INST] «SYS» {system_level_instruction} «/SYS»{instruction}[/INST]", where parameter
system_level_instruction sets desired behaviour constraints (e.g. tone, response style), and parameter
instruction specifies task (see the caption of Table
9 (Appendix
A), for an example). SFT was conducted with the same parameters as in Table
3, except for the learning rate, which was set to 0.00001, and the context length was restored to 4096.
Hyperparameters, such as learning rate, warmup ratio, weight decay provided in Table
3 were selected according to Touvron
et al. (
2023) guidelines, but slightly adjusting the values provided to ensure faster and more stable loss minimisation. During pretraining we also observed gradient exploding effects. We mitigated them by tuning gradient accumulation steps (see Table
3).
Table
10 (Appendix
A) provides text generation examples (pretrained models), and Table
9 (Appendix
A) provides examples of answers to questions (pretrained and fine-tuned models). If not stated otherwise, in all experiments and benchmarks with the proposed LLMs, we used pretrained-only models, which corresponds to the common practice. The download links for the proposed LLMs are provided in Table
8 (Appendix
A).
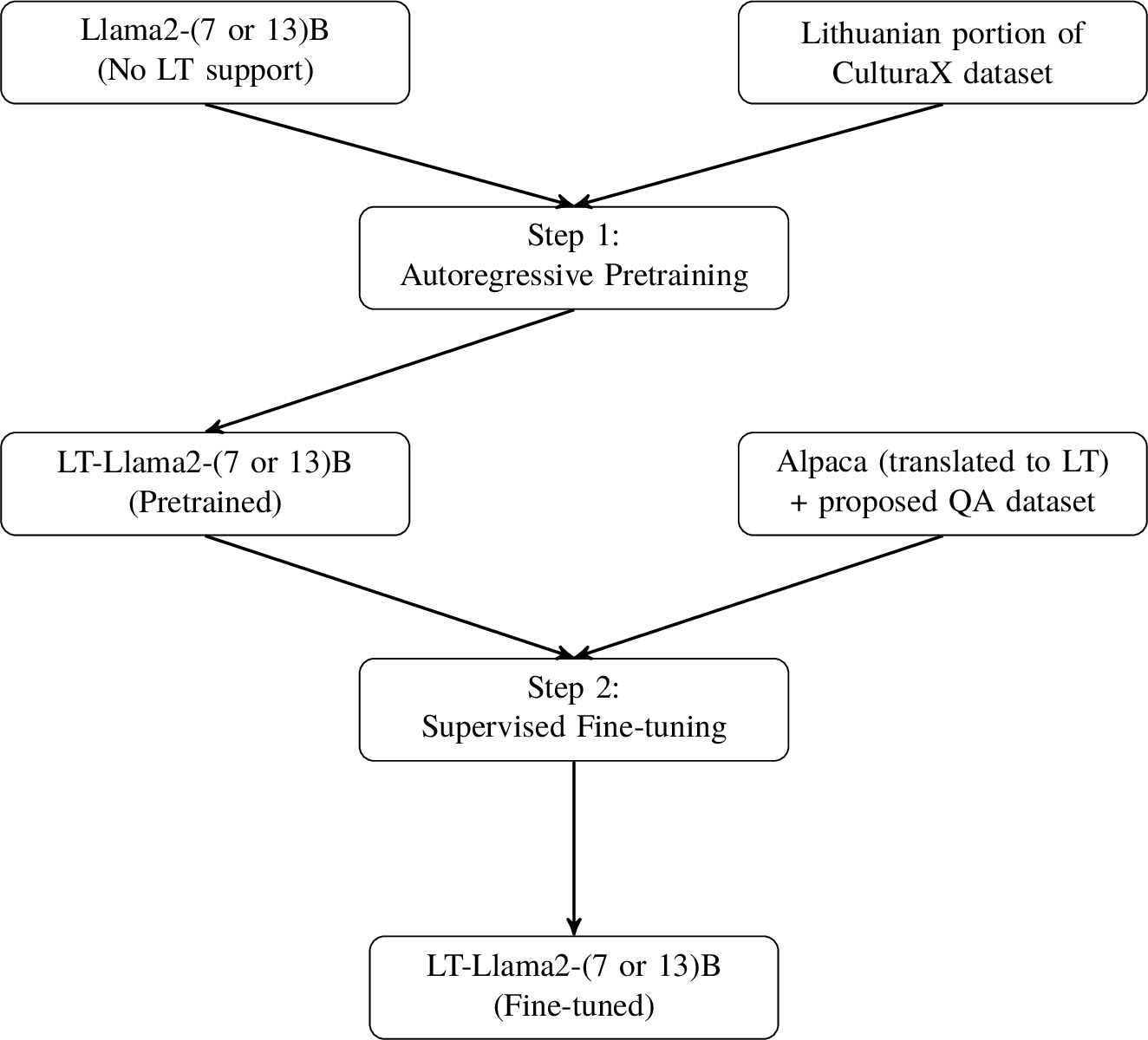
Fig. 1
Overview of the two-step process for creating LT-Llama2-7B/LT-Llama2-13B.
Table 3
Hyperparameters and other details.
Learning parameter |
Llama2-7B |
Llama2-13B |
Number of epochs |
1 |
1 |
Learning rate |
0.0002 |
0.00004 |
Warmup ratio |
0.05 |
0.05 |
Weight decay |
0.07 |
0.05 |
Per-device batch size |
8 |
4 |
Gradient accumulation steps |
2 |
4 |
Duration of pretraining in hours for a single H100 GPU |
1722.0 |
2980.5 |
Duration of fine-tuning in hours for a single H100 GPU |
$\lt 1$ |
$\lt 1$ |
Total number of tokens |
14761219995 |
Records in dataset |
13339785 |
Mean number of tokens per record |
1106.5560 |
Standard deviation of tokens per record |
697.0089 |
Optimiser |
AdamW |
Hardware |
8xH100 GPUs |
Proposed open Q/A dataset. This dataset was constructed from the ChatGPT (OpenAI
et al.,
2024) summarisations of a subset of Lithuanian Wikipedia using the procedure described below. First, the Lithuanian Wikipedia was downloaded and the titles of its pages were filtered with the following prompt:
"I will provide a list of titles in Lithuanian language. From the list provide me the titles without any explanation which are directly or indirectly related with Lithuania except fauna and flora. List: {list}", where variable
list represents a list of titles of all pages. After this filtering and manual check, the resulting list of Lithuanian Wikipedia pages represented by pair (
title,
text) was transformed into Q/A pairs using the prompt, returned by Algorithm
1.
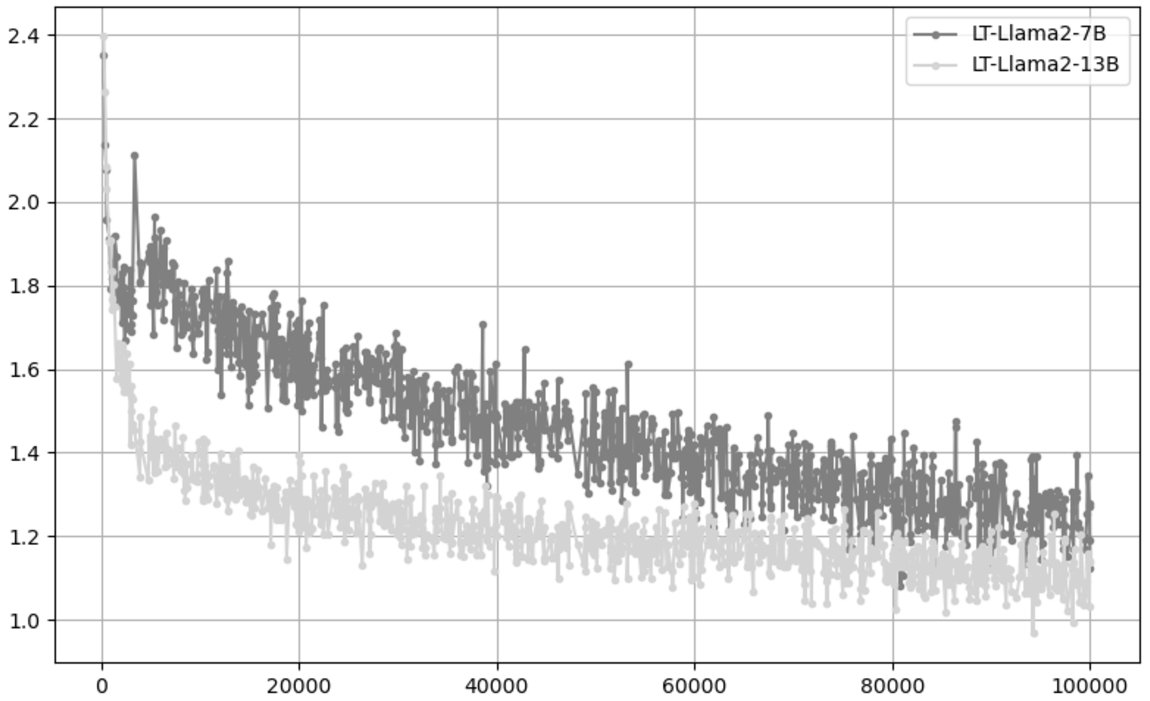
Fig. 2
Losses (y-axis) vs training steps (x-axis) during the model’s pretraining.
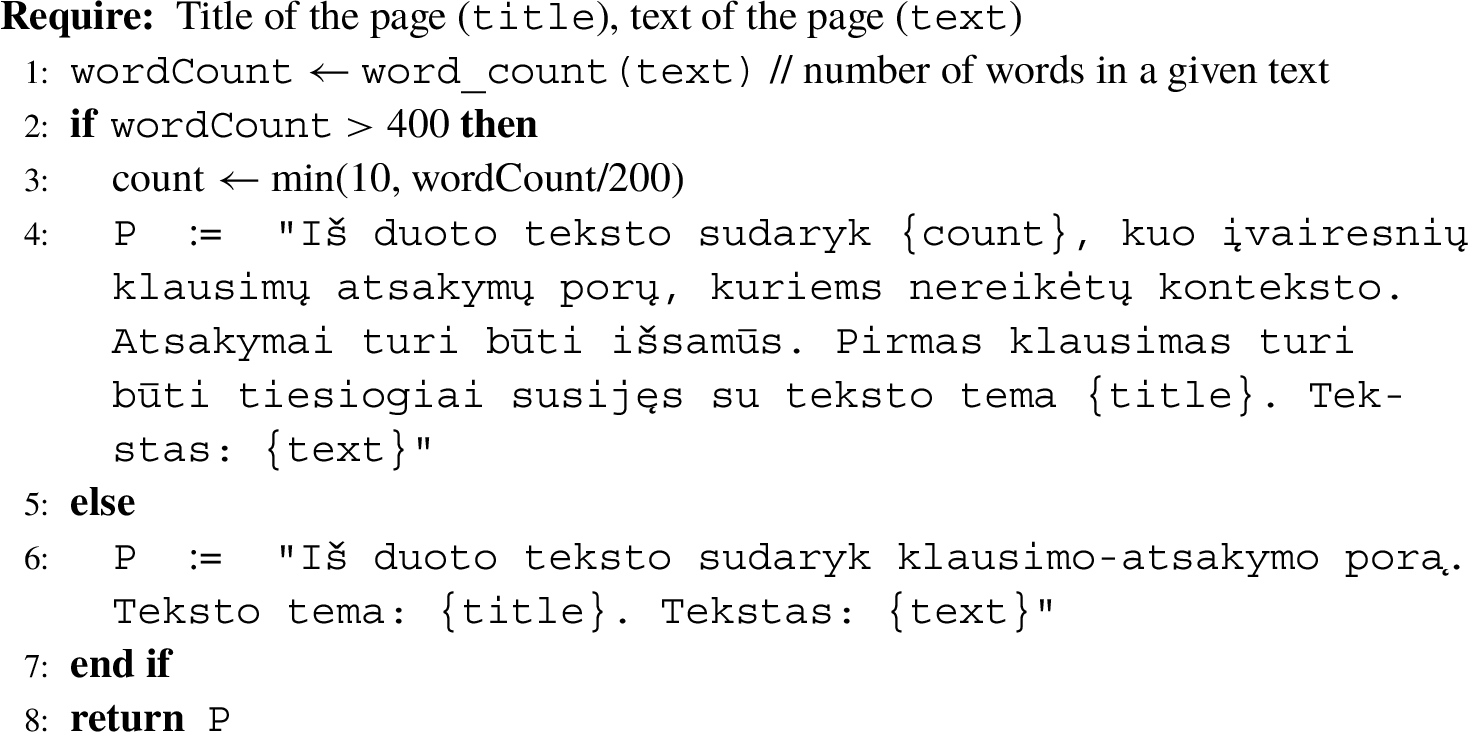
Algorithm 1
Generate prompt for Q/A summarisation
The proposed dataset consists of 13,848 such pairs, and represents various facts about Lithuania and Lithuanian history. Note that it was not used in the pretraining process. Table
4 presents a set of examples from the proposed Q/A dataset, which can be accessed through the download links provided in Table
8 (Appendix
A).
Proposed open translations of language understanding benchmarks. Language model evaluation harness (LMEH, Gao
et al.,
2023) are language understanding benchmarks which are created for the evaluation of LLMs across a wide range of tasks. LMEH includes a set of popular LLM evaluation benchmarks:
Table 4
Examples from the accompanying Q/A dataset.
Question |
Answer |
Koks yra Vilniaus miesto statusas Lietuvoje? |
Vilnius yra Lietuvos sostinė. |
Kur yra Gedimino pilis? |
Gedimino pilis yra Vilniuje, ant Gedimino kalno. |
Kas buvo vadinamas „Lito tėvu“? |
Vladas Jurgutis buvo vadinamas „Lito tėvu“, nes jam buvo patikėta spręsti visus naujos valiutos įvedimo niuansus. |
Kokios upės teka per Vilnių? |
Per Vilnių teka Neris ir Vilnia. |
Kada buvo įkurtas Vilniaus universitetas? |
Vilniaus universitetas buvo įkurtas 1579 metais, Vilniuje, po Lietuvos didžiojo kunigaikščio Stepono Batoro privilegijos suteikimo jėzuitų ordino kolegijai. |
Kada ir kur įvyko Žalgirio mūšis? |
Žalgirio mūšis įvyko 1410 m. liepos 15 d. netoli Tanenbergo ir Griunvaldo (Žalgirio) kaimelių, dabartinės Lenkijos teritorijoje, į pietvakarius nuo Olštyno. |
-
• Arc (Lai
et al.,
2023) benchmark consists of multiple choice science questions at school level.
-
• GSM8K (Cobbe
et al.,
2021) benchmark consists of linguistically diverse mathematical problems.
-
• Hellaswag (Zellers
et al.,
2019) benchmark consists of common-sense inference challenge dataset.
-
• Massive multitask language understanding (MMLU) (Hendrycks
et al.,
2021) benchmark covers different tasks from a diverse set of academic disciplines and is designed to measure the accuracy of the model in a multitask setting.
-
• Truthful-qa (Lai
et al.,
2023) benchmark is designed to measure whether an LLM is truthful in generating answers to questions that span different categories (health, law, finance, and politics).
-
• Winogrande (Sakaguchi
et al.,
2019) is a set of pronoun resolution problems originally designed to be unsolvable for statistical models that are based on selectional preferences or word associations.
These benchmarks produce prompts consisting of question and answer options, and evaluate the accuracy of the responses of LLMs. The accuracy can be measured conveniently because of the structured prompt, which asks the LLM to select an option (e.g. "a", "b" or "c"). We translated the LMEH benchmarks into Lithuanian using GPT-4. The download links are provided in Table
8 (Appendix
A).
5 Conclusions
We presented the first Llama2-based open LLMs tailored especially for the Lithuanian language, the accompanying Q/A dataset, and the translated LMEH benchmarks, which contribute to the standardisation of the evaluation of Lithuanian language models.
We also provided an overview of the existing LLMs for common European languages. It shows that most regional models follow the Llama2 or Mistral architecture. In addition, some authors do not train a full parameter set, but instead rely on PEFT approaches, which are less computationally demanding but also potentially less efficient in performance. On the other hand, PEFT methods partially allow one to retain the original parameter structure, and thereby they may be beneficial for achieving more efficient regional LLMs from the perspective of language understanding benchmarks. Our findings also reveal a lack of scientific documentation of the published open regional LLMs.
We evaluated the proposed LLMs based on perplexity and translated LMEH benchmarks. During the pretraining epoch, we evaluated average perplexities (measured with independent dataset) every
$10\% $ of the training iterations. These benchmarks show that perplexity decreases consistently during pretraining, reflecting enhanced next-token prediction capabilities. The initial and final perplexities (17.4613 versus 3.8096 for LT-Llama2-7B and 13.8849 versus 3.4520 for LT-Llama2-13B) show the integration of the Lithuanian language component in the proposed Llama2 models. Using the same scheme, we also evaluated our models with the translated LMEH set, which includes a conceptually diverse set of language model benchmarks. The results of these experiments hint that the Lithuanian component of CulturaX may not be sufficiently rich for modern LLM architectures. Although we positively answer the question of whether efficient Lithuanian LLMs (which were non-existent at the beginning and during most of this research) can be achieved from Llama2 LLMs, which lack Lithuanian components, the latest open multilingual models (Llama3.1, Llama3.2, Gemma2, and EuroLLM) already have a strong Lithuanian component. According to our benchmarks, these open SOTA LLMs generally performed better than our models, however, the proposed LT-Llama2-13B was ranked average (
$4/8$) in half of the LMEH benchmarks. This also leads to the hypothesis that by deriving Lithuanian LLMs from these recent models, one may obtain more efficient Lithuanian LLMs. In our opinion, the good performance of our model in the external benchmark by Kapočiūtė-Dzikienė
et al. (
2025) may be due to the fact that it was trained in a single language and the other LLMs were multilingual.
In the context of regional LLMs, the proposed models open up further research perspectives not only for NLP, but also for other directions, since LLM representations are potentially useful in various scenarios (e.g. sentiment analysis (Zhang
et al.,
2024), robotics (Kim
et al.,
2024)). The important limitations of our contribution are related to the rapid progress of LLM research, leading to the continuous emergence of more advanced models. In addition, we used automatically translated and generated data in the contributed components, which may also cause negative effects. To achieve stable loss minimisation during pretraining we faced and solved several challenges related to the selection of hyperparameters (learning rates, batch size, gradient accumulation steps). Our future work will include fully trained small language models tailored for Baltic languages and English.